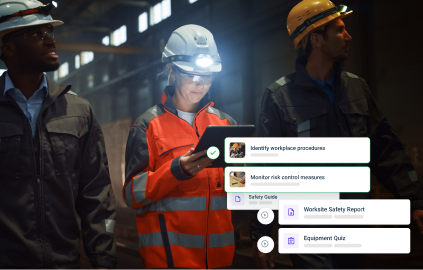

How your RTO can optimise Content Management and Assessment Authoring for maximum efficiency
In the dynamic landscape of education and training, the creation and delivery of quality assessments play a pivotal role in the credibility of training organisations.